Zhuge Liang said: "To reap the benefits, one must first understand the drawbacks," and this applies to any new technology. To effectively utilize AI, it is imperative to recognize its shortcomings. The drawbacks of AI are quite straightforward: it has low reliability and can only be used in contexts where reliability requirements are not stringent. Given that the strengths of AI lie in its extensive knowledge and powerful computational abilities, it is best suited for applications where reliability is less critical, but knowledge and computational capacity are highly valued. Based on this principle, the scenarios for the application of large industrial models become clearer.
01
I originally did not intend to write this article, but I couldn't resist. The reason for not wanting to write it was that I felt my understanding of large industrial models was superficial and not worth discussing; the urge to write came from seeing some people making nonsensical statements, which I couldn't help but refute.
I acknowledge the immense role of large models, and as technology advances, their role will become even greater. Last year, my friend Xiao Hou tried a foreign model and remarked with a sigh: the capabilities of large models are roughly on par with those of a master's degree student. In the future, engineers who do not use large models may become obsolete, just like people today who do not know how to use the internet. There is a fascinating saying: the AI of the future may not necessarily defeat humans, but those who know how to use AI will defeat those who do not.
Advertisement
02
Zhuge Liang said: "To reap the benefits, one must first understand the drawbacks," and this applies to any new technology. To effectively utilize AI, it is imperative to recognize its shortcomings. The drawbacks of AI are quite straightforward: it has low reliability and can only be used in contexts where reliability requirements are not stringent. Given that the strengths of AI lie in its extensive knowledge and powerful computational abilities, it is best suited for applications where reliability is less critical, but knowledge and computational capacity are highly valued. Xiao Hou said: the essence of a large model is an "assisted thinking system": what you might not think of, the large model might consider. There is a possibility that the model could be wrong. What should one do? Let humans be the gatekeepers!
Based on this principle, the scenarios for the application of large industrial models become clearer: they have great potential in places that rely on human decision-making; in places where decisions are automated, a case-by-case analysis is required. I do not oppose the use of AI for automated decision-making. On the contrary, I have always emphasized that AI, especially image recognition, has a breakthrough role in advancing automation technology. However, in some other contexts where decisions are automated, the application of AI should be approached with caution. For instance, it has long been emphasized by humanity: do not allow machines to kill autonomously.Management, research and development, service, maintenance, and other processes rely on human decision-making. Therefore, large models can be used to assist people in their work in research and development, service, and other areas. For example, when designing products, large models can help people come up with several optional plans, identify issues with specific plans, and provide warnings; in terms of service, they can help users choose products and product combinations. In these application scenarios, humans are the ultimate decision-makers. Large models act as consultants and strategists.
However, caution is needed in the field of production automation. For instance, I do not advocate for large models to automatically optimize key process parameters that significantly affect quality. Why? Because these parameters often belong to corporate standards and are the result of practice, and they should not be arbitrarily altered.
Many people do not understand my viewpoint. To grasp this perspective, one must understand the nature of industry, especially modern industry. I discussed some industrial common sense in "Zhi Xing" (a book or article), and many people do not know why I talked about it. This is a good opportunity to explain.
In the book, I mentioned that one of the characteristics of modern industry is the high quality requirements. When the quality reaches a certain level, the concept of "broad quality" comes into play. Broad quality not only demands that the physical quality of the product be high but also requires a high degree of standardization in the production process. Why not directly measure quality using product quality indicators? There are several reasons: First, the quality of industrial products is not only about the high quality indicators of the product itself, but also the stability between these quality indicators is very important. Second, some quality indicators are unclear or difficult to measure universally. Therefore, when product quality requirements are particularly high, the stability of raw materials and the process must ensure the quality requirements. Hence, the production process often needs to be strictly carried out according to standard process requirements.So, even if the quality indicators of the product itself are better, if the indicators are unstable, it is also poor quality, and unstable process parameters are also poor quality. Understanding this principle, we understand that process parameters must be executed according to the standards set during the research and development process, and it is not permissible for industrial large models to automatically provide so-called "better practices."
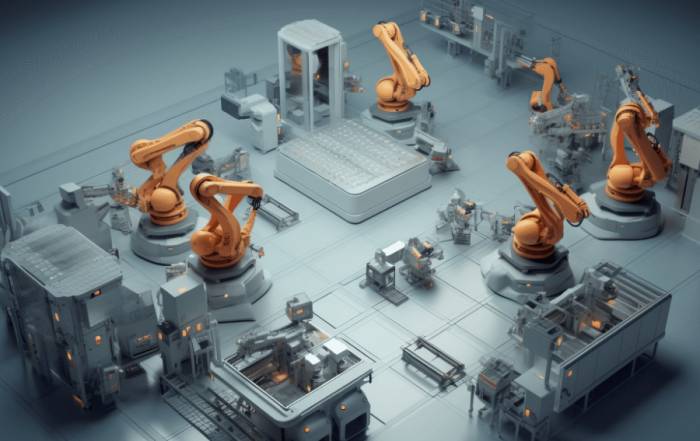
This topic can be elaborated further: Can process parameters be dynamically optimized? In fact, it is also possible. But the premise is: The principles of dynamic optimization must be standardized, and they must be clearly stated. In other words: Changes to the standard must conform to the standard. Therefore, even if the industrial large model is superior, its application is often non-standard: because it is difficult to clearly state. In addition, the optimization of process standardization is generally aimed at a specific working point. At this time, the optimization function can often be approximated by a linear function, and there is no need for a large model at all.
04
Many people have a shallow understanding of the standardization in modern industry. We must recognize that even if your approach is more effective, it is not good to violate the rules, and this is the characteristic of modern industry. This is very similar to the thinking of modern law: you may be right, but if there is no evidence in court, you will be judged to lose according to the law. Only by learning to respect the rules can we understand modern industry.
Comment